Top Most Data Labeling Challenges in Annotation Companies
By Anolytics | 13 October, 2020 in Data Annotation | 5 mins read
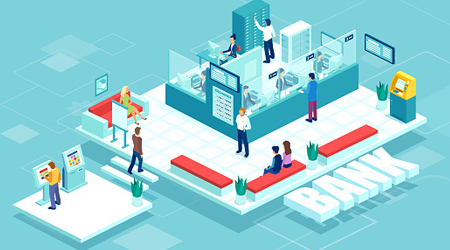
Data labeling is not a task, it requires lots of skills, knowledge and lots of effort to label the data for machine learning training. And for visual perception model needs annotated images to train the computer vision algorithm helping the model to recognize the various objects recognizable.
However, while labeling the different types of data companies encounter various problems making the labeling tasks more time taking and ineffective. To make the data labeling more effective and productive we need to understand these problems. So, in this blog post, we will discuss data labeling challenges with few suggestions to overcome such problems.
Top 5 Data Labeling Challenges
#1 Managing the Team of Large Workforce
To manually annotate the images of label data, you need a huge amount of workforce that can generate a massive volume of training data for different types of machine learning models. Actually, machine or deep learning needs a huge quantity of datasets and to manage such data managing a team of large team workers is also a very challenging task.
Also Read: Why Human Annotated Datasets is Important for Machine Learning
Actually, merely generating the data is not enough, maintaining quality is also important to produce high-quality training data for deep learning models. And while dealing with data labelers you need to face the following listed problems.
- Training the New Data Labelers for Different Tasks.
- Distribution of work flawlessly across the team and assigning them the tasks.
- Checking and solving the technical issues faced by the labelers.
- Ensuring the communication and collaboration between the labelers.
- Checking the quality control and validating the data sets.
- Overcoming the cultural, geographic, and language barriers between the labelers.
#2 Ensuring the Quality of Data with Consistency
If the quality of data is not at par, a machine learning model will not get trained with the right inputs, resulting in the predictions made by AI model would be not right. Hence, producing high-quality training data is another challenge for data annotation companies.
And merely producing the quality training data but producing high-quality data with consistency is also equally important to make sure the right predictions by the AI model. There are two main types of dataset quality — subjective and objective — and they can both create data quality issues.
Also Read: How To Improve Machine Learning Model Performance: Five Ways
Subjective Data: Labelers have different cultural values, expertize and language or geographical backgrounds that can influence the way they interpret the datasets. Actually, there is no single source of truth it is difficult to define the label in such situations.
Let’s take an example, if labelers are shown, there is no conclusive answer to whether given a video scene is funny. Based on their own biases, personal history, and culture; the labeler might give a different answer when they repeat the task in the future.
Objective Data: While on the other hand, if data is objective, and the answer is no single, then again challenging is ahead there. Actually, at the initial stage, there’s a risk that the labeler might not have the domain expertise needed to answer the question correctly.
To understand such a situation better let’s take an example. When labeling leaves, will they become knowledgeable enough to recognize them as healthy or diseased? Moreover, without good directions, labelers might not know how to label each piece of data, like whether a car should be labeled as a single entity “car,” or if each part of the car should be labeled separately.
Lastly, it’s impossible to eliminate the errors dome by these humans’ means annotators, no matter how good your dataset quality verification system. Such a situation leaves the data annotation team finding another way to resolve the subjective as well as objective data quality issues. And it is possible if they can set up the closed-loop feedback process to check the errors regularly.
Also Read: How To Ensure Quality of Training Data for Your AI or Machine Learning Projects
#3 Selecting the Right Tools & Techniques
To generate the high-quality training datasets combination of well-trained workers and the right tools is very important for the data annotation companies. Though, automated machines or AI-assisted data labeling or manual data annotation or automation and data management all need to understand.
Actually, depending on the types of data, different types of tools and techniques are used to label the data for deep machine learning. There are different types of tools and software available in the market specially developed for data labeling. Bounding box annotation, semantic segmentation and point cloud annotation are the leading image annotation techniques considered while labeling the data.
Though, in-house tooling demands a great extend to invest in developing such customize tools. And in manual data labeling, some companies go with a conservative approach that makes it difficult if not capable enough to meet the data labeling requirements.
Actually, building your tool does not only increase your cost but also affect the quality of the datasets. Hence, when it comes to buying the tool from a third party, you need to consider whether the tools you select provide all the services that you’re looking for. Here it becomes critical to choose the robust data annotation platform that can ensure the quality and available at affordable pricing.
#4 Controlling the Cost of Data Labeling
Acquisition of training data is one the important factor accounts for the major cost of AI project developments. And most of the AI companies struggler due to low budget making their data labeling needs indispensable, especially there are requirements of a huge quantity of datasets.
We’ve often noted a lack of transparency into exactly what enterprises are paying for in their data labeling projects, whether it’s in-house or contracted out. And organizations that outsource data labeling generally need to choose between paying for data labeling per hour, or per task.
Also Read: Five Reasons Why You Need To Outsource Your Data Annotation Project
Paying per task is more cost-effective, but it incentivizes rushed work as labelers try to get more tasks done in a given timeframe. However, most enterprises prefer to pay per hour. For small business houses, manual data labeling teams run very expansively owing to the time and training to achieve expertise.
#5 Complying with Data Security Standards
Complying with international data security standards like GDPR, CCPA and SOC2 or DPA are one of the challenge data annotation companies’ face. Data confidentiality compliance regulations are increasing globally as more organizations gathering more and more data.
In fact, when it comes to labeling unstructured data, this includes personal data such as faces; reading the text and any other identifying data appearing in the images. Data labeling companies are obligated to comply with internal data security and privacy standards.
Also Read: Why Outsourcing Your Data Annotation Needs to GDPR, CCPA or SOC Type Compliant Companies
And while companies to data security standards, companies have to ensure that their data is secure, prevented workers from accessing it using any insecure device, downloading and transferring it to an unknown storage location, or working on data in a public location where it could be misused by someone without security clearance.
Creating such a highly secured environment is a challenging tasks for data labeling companies. As while outsourcing the data labeling tasks annotation companies must comply to process the highly sensitive data to keep it safe and protected until it is delivered to the clients.
Considering all such data security measures, the clients of Anolytics trust in them to deliver high-quality training data to them with complete security and we do so by achieving the best security practice implemented everywhere – we can do from virtual to physical tech spaces.
And Anolytics is providing the image annotation services with the ability to overcome all the challenges. It is working with a team of well-trained skilled annotators and using the best tools or techniques to the developer the high-quality training data developed in a highly secured environment to ensure the success of the AI model that can work in various scenarios with the best level of accuracy.
please contact our expert.
Talk to an Expert →
You might be interested
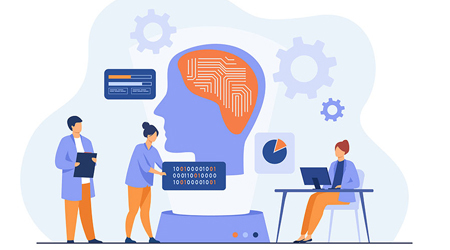
- Data Annotation 02 Aug, 2019
Five Reasons to Outsource Your Data Annotation Project
Artificial Intelligence (AI) and Machine Learning (ML) development is mainly rely on training data sets, that helps the
Read More →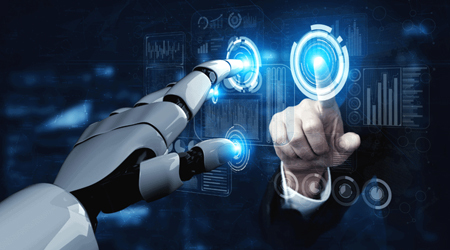
- Data Annotation 17 Dec, 2019
Why Data Annotation is Important for Machine Learning and AI?
Data annotation is the process of making the contents available in various formats like text, videos and images, recogni
Read More →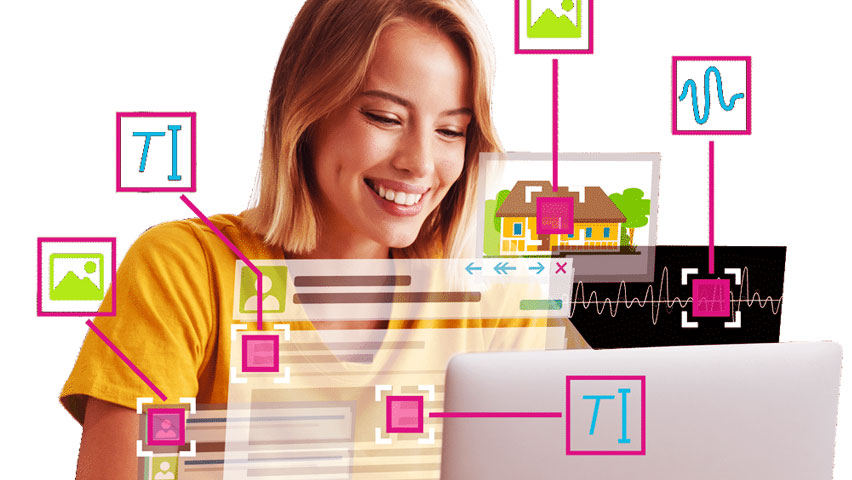
- Data Annotation 25 Mar, 2022
What is Data Annotation and it’s importance?
Data annotation is the process of labeling the data available in various formats like text, video or images. For supervi
Read More →