Complete Guide to AI & Machine Learning in Retail: Types & Use Cases
By Anolytics | 28 March, 2023 in AI in Retail | 10 mins read
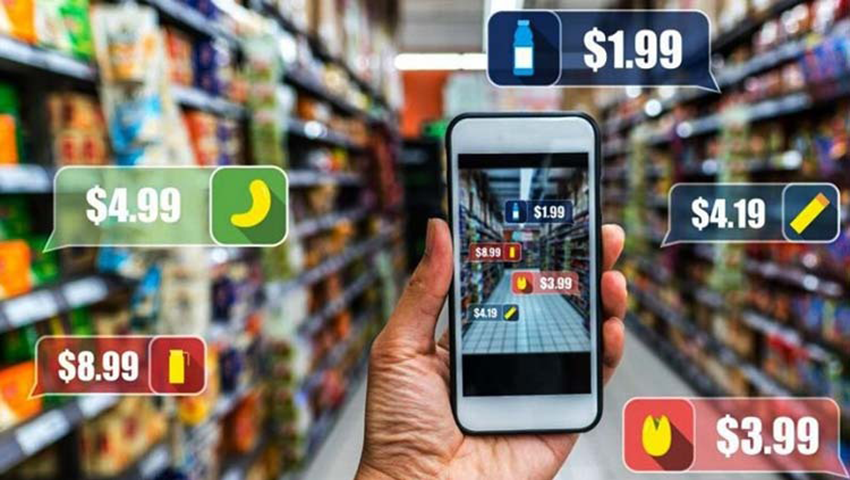
We have stepped into an era, where Machine learning (ML) and artificial intelligence (AI) play a huge role in the retail industry, especially for those that heavily rely on online sales. AI has been successfully integrated by big players such as eBay, Amazon and Alibaba throughout the entire sales cycle. Machine Learning prove to be beneficial to businesses irrespective of how big or whether they sell products exclusively online.
This guide talks about the best ways in which online retailers and brick-and-mortar physical stores of any size can use Machine Learning in retail to increase overall sales and reduce overhead costs.
Clothing, groceries, and household items are just a few of the items that are found in retail spaces. A fraction of the possible Machine Learning in Retail projects are presented in this guide. The intention behind developing this guide is to showcase the limitless possibilities of Machine Learning in Retail sector.
Strategies for Setting Prices Right
Retail and e-commerce businesses must know how to price an item and scale pricing operations intelligently, consistently, and efficiently. Pricing decisions are dramatically improved with artificial intelligence and data intelligence.
Pricing by Auto-Adjustment
At times, it is a general tendency for people to define an item’s price based on how they personally value it. Platforms for resale and shopping usually follow the same pattern. As different people value the same item differently, this manual process sometimes leads to inconsistent results. A human bottleneck also affects publication or upload speed and costs: every piece published on a site needs to be priced by a human. Also, it is quite difficult to remember the numerous factors that influence product demand and price, directly or indirectly. The most efficient way to go about it is to automatically and consistently price every new item by looking at data using image recognition technology. An AI model leveraging image recognition technology analyzes the appearance of the product and considers other factors such as brand, condition, fabric, category, description, sales data, and past pricing decisions. The optimal price is calculated based on these data points. Furthermore, there are options to include competitor pricing if it is available, as well as many other features.
AI models automate the vast majority of pricing operations. Thus, helping businesses scale to more items effortlessly.
Optimizing Prices
Profitability is strongly influenced by pricing. With the help of Machine Learning algorithms, it is possible to define an automatic pricing strategy that incorporates key pricing variables in real-time.
Customer behavior can be used to estimate willingness to pay, such as the items the customer looks at and purchases, or the length of time they spend on each web page.
In order to maximize profits, marketers must understand how clients respond to products at different price points and estimate the optimal price for each product.
Applying machine learning in retail can make the life of business owners and marketers easier. Dynamic price adjustments are regularly advised based on a forecast of demand and consideration of many factors. Automatic pricing systems can detect the most profitable or estimated “optimal” price by considering supply, seasonality, external events related to your business (such as concerts, sporting events, festivals), and market demand.
To train such a system or AI & ML model one requires historical sales data, but unlike auto-pricing, past prices differ for each product, which makes the system more accurate. In order to estimate the price-demand relation (usually called demand elasticity), it requires “exploration”. The training dataset for the retail industry is extremely important because biased or inaccurate training data will lead to inaccurate results.
Assume that past prices have not fluctuated. In such a case, iterations are mostly in “exploration mode,” involving introducing some randomness to prices and observing how sales data react.
As the system gains knowledge about what direction to move in each case, it can gradually expand the range, without significant deviations from previous prices.
Pricing strategies have a lot of room for improvement, and the benefits can be felt by both sellers and customers, since low prices can lead to a surprising increase in profitability in most cases.
Choosing the Right Product
The process of matching products between two or more catalogs involves identifying and linking identical items.
In addition to price comparison with competitors or different providers of the same products, you can merge multiple offers from different sellers into a single product page (for instance, Amazon’s buy box), as well as perform assortment gap analysis against your competitors, among other less obvious uses.
We can better understand the business value of solving this problem by looking at the example mentioned. Ninety-six percent of retail customers compare prices before buying. Any business can track their competitors’ prices by using product matching.
Large and messy product catalogs become increasingly common as businesses grow and expand. Sometimes, products have codes that uniquely identify them, making matching trivial. However, these codes are rarely available or sellers do not disclose them. Even the codes are sometimes incorrect! It is also possible that the products may be the same, but their presentation may differ. As an example, a single bottle vs. a case of six.
All of this leaves us with a complex problem: how to match the products using the information available? Read on. Images, free-text descriptions, other metadata, and product titles can all be included here.
When catalogs grow in size, this matching task escalates out of control fairly quickly. When you match multiple catalogs (separate competitors or suppliers), it gets even worse.
How can this process be made easier? An AI solution that compares each product’s image or unstructured text fields, such as title, attributes, and description, to find the most similar pairs. There is a human-in-the-loop process to verify suggested matches.
• Using the first batch of manually verified matches, the system is retrained (using them as examples of verified matches).
• For each type of product, AI learns which features to use.
• For the remaining items, we can perform a second iteration and the suggested matches will be more reliable.
• The system is being trained again.
This process can be repeated according to the level of confidence and needs of a particular problem.
Marketing Campaign Optimization
The marketing campaign, like pricing strategy, plays a crucial role in retail business, but it is also complex and requires a deep understanding of market factors. Machine Learning models enhance decision-making by forecasting ROI and providing optimal parameters for its execution based on historical data. Model predictions are used by decision-makers to balance costs and profits when designing campaigns.
When designing Marketing Campaigns, AI models can target specific business metrics such as net revenue or margin contribution. The use of Machine Learning to optimize Product Promotion Campaigns allows Marketing Teams to leverage their creativity and experience more effectively.
Product Replenishment optimization (recommend what to buy for clients in b2b2c)
By using specialized recommendations, Product Replenishment Campaigns aim to increase repeat purchases by retailers, especially B2B2C businesses. A successful campaign requires a thorough analysis of the market and a deep understanding of the target audience.
Predicting customer behavior
In a predicting customer behavior system, previous behavior is used to predict how buyers will behave in the future. Personalized marketing actions can be performed more effectively using these systems than general marketing approaches. Furthermore, customer loyalty and retention are increased when actions are taken according to predicted customer needs.
For example, to determine which customers are likely to make a purchase within a week and predict marriage or pregnancy, and then send custom offers.
It is basically necessary to collect data on consumer behavior in order to build predictive AI models. In other words, it could include purchases history, buying trends, and social media activity. The ability to predict consumer needs is a challenging task that Machine Learning algorithms can help with.
• What is the average number of transactions your customers make?
• Do they purchase during sales time or before their date of birth?
• What is the average number of product items they buy?
• What are they buying at the moment?
• What topics do they discuss on social media?
• Models use this type of information to predict future behavior.
Finally, it is very important to fine tune AI models based on retailer experience.
Retail Stocking and Inventory
The optimization of inventory planning and predictive maintenance is a critical logistical concern for retailers. Moreover, if an e-commerce platform fails to fulfill an order due to out-of-stock products, the UX of end users could be adversely affected. Using machine learning in retail, retailers can keep a track of the inventory and never run out of stock.
Predicting inventory
Real-time inventory needs can be predicted by machine learning algorithms using purchase data. These algorithms can provide a daily dashboard of suggested orders based on the day of the week, the season, nearby events, and social media data.
In scenarios where e-commerce platforms do not manage inventories directly, we have built similar solutions to predict whether an item a user is buying might be out of stock. In order to develop a predictive model, historical data is utilized, such as past stockout events, demand, price, and stock level for each product. Predictions are also made based on real-time data such as price updates and date-time features. Users would be informed in a timely manner if their items were out of stock by e-commerce platforms.
Consumer behavior data is needed for inventory planning models. Purchasing history or trends are examples, but social media activity and domain knowledge can also be included.
It is also possible to leverage the algorithms used for pricing optimization for stock management, because they need a sales forecasting model (as a function of price) to determine the optimal price. They usually work together so that early out-of-stock events are avoided (because they are always a suboptimal solution).
e-Commerce
Tagging and copywriting automation
When a site is crowded, searching for items can be challenging. Retail teams spend days describing items and tagging them according to their primary attributes and preferred taxonomy. In the past, this has been a manual process that relied on each person’s time and criteria, resulting in inconsistent data.
Traditional manual processes have a few of the following issues:
• There is an inconsistency in the descriptions of similar items (some are too detailed and long, some are too brief).
• Missing attributes
• Criteria for specific attributes or values are not aligned between humans
An AI solution uses computer vision technology to automate item tagging and descriptions, obtaining consistent and standardized data. The information comes directly from the image. Automatically, the machine fills in product attributes and tags them accordingly to place them in the correct category and hierarchy on the website. It is as simple as uploading the photo. Depending on each retailer’s taxonomy, this can be adjusted.
Image retouching
Images help online shoppers understand product features as fully as possible. Photos that look professional can enhance an e-commerce site, making customers more likely to make a purchase and brands more satisfied with how their products are presented.
Usually, pictures are taken in a photo studio and then sent out for retouching. Depending on each site, retouching involves several steps. The most common ones include calibrating colors for accurate depiction (you don’t want customers returning items because the colors don’t match their expectations! ), removing backgrounds, aligning/rotating items, smoothing creases, and erasing mannequins. The whole process of uploading an item can take several days due to this delay.
The majority of this time-consuming process is better to be automated with artificial intelligence to cut corners. AI based automated image retouching models helps reduce both time and costs on a large scale. This system replicates the traditional “Photoshop pipeline” used by artists but on a much larger scale. Using artificial intelligence and machine learning in retail hundreds of thousands of images can retouched in just a matter of a few minutes.
Browsing experience
The process of browsing online catalogs can be exhausting like searching for a needle in a haystack, or it can be a rewarding experience that teaches you a lot about the products you’re looking for. It is largely dependent on how relevant the results are that the system provides when you interact with it. A search term does not suffice to solve the problem of Information Retrieval in computer science. Generally, we can think of it as: provided a query, provide the most relevant results, usually defined as those which the user is most likely to click, so it’s evaluated by measuring click-through rate.
Visual search
Visual content is often searched for by customers before making a purchase. It can be difficult to find good keywords for what they want in some cases. By using visual search, consumers will be able to find what they are looking for much more easily.
Uploading an image instead of typing a query such as ‘an elephant shaped pillow in purple colour’ can help narrow down the search down to more specific items. It is currently possible to achieve amazing results through Machine Learning algorithms with the huge amount of snapping and sharing of images.
Recommendation engines
Retail and e-commerce companies are using recommender systems on their websites to boost sales by leveraging data.
Companies that use recommender systems focus on increasing sales by providing highly personalized offers and enhancing customer service. Users can access content they’re interested in quicker and more easily through recommendations, and they can be surprised with offers they would not have found otherwise.
Companies can also gain and retain customers by sending out emails with offers and suggestions that match recipients’ interests.
The chances of users buying add-on products when they get a sense of familiarity. When businesses are aware of what their users want and showcase it to them without delay, the probability of them leaving is less.
Incorporating recommendations into products and systems adds value to users. A competitive advantage also allows businesses to earn more money by positioning themselves ahead of their competitors.
Counting of Human flow in Physical stores
Retailers can measure human flow in stores to determine how effective their stores are at converting customers, as well as the number of people entering their stores throughout the day. Optimizing operational resources can improve customer service.
Based on pre-installed security cameras, retailers can built a video analytics solution that counts people entering and exiting stores based on computer vision algorithms. Using Machine Learning in Retail. retailers will be able to gain greater insight into their business by leveraging their existing CCTV infrastructure.
Analyzing navigational routes
Physical retailers strive to understand the customer’s path to purchase by deciding where to place different items.
To understand how customers interact with stores, computer vision algorithms can track their journeys. Algorithms can detect walking patterns and gaze directions of customers. In addition to reorganizing store layouts or measuring interest in products, retailers can use this information to restructure their store layouts. It would help them discover locations or spots that get maximum traffic and/or visual attention.
For example,
• What proportion of teenagers cover a store’s front part, for instance?
• Does the store get more traffic in the winter?
• Product placements and promotions could be dynamically modified using variables such as age, day of the week, or season.
Tracking products and recognizing gestures
In brick-and-mortar stores, most items are picked up, glanced at, and then returned. The company or owners hardly get to have any information about what the next step is for the customers.
Using computer vision, shoppers can estimate an item’s interest level based on their facial and hand gestures. In these applications, valuable data is generated about how many times an item is picked up, purchased, and returned.
Theft prevention
Detecting shoplifters using Machine Learning technologies can go beyond the typical use of video cameras in retail theft prevention. It is possible to train facial recognition algorithms to detect shoplifters when they enter the store. This technology was tested by Walmart in 2015 as an anti-theft mechanism. Computer vision can also detect when someone hides an item in their backpack or jacket, in the same way it can detect when someone picks up an item. Furthermore, the same approach can be used to detect checkout clerks who skip scanning items intentionally or unintentionally.
Security personnel or managers can be alerted in real-time and sent video excerpts to judge the situation by themselves before confronting the individual.
Final Thoughts
In an ever-evolving consumer paradigm, retail continues to grow and face new challenges. A company’s ability to serve these necessities with state-of-the-art technology can determine whether it succeeds or fails.
At Anolytics, we help retailers leverage the power of AI and ML models by providing highly refined training dataset for retail industry.
Get in touch with us if you’re thinking of integrating AI and ML into your retail business strategy.
please contact our expert.
Talk to an Expert →
You might be interested
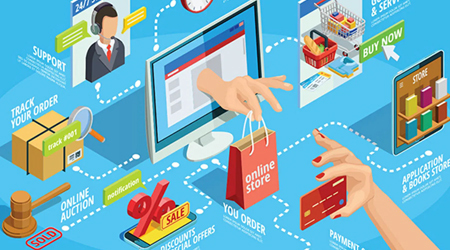
- AI in Retail 14 Jan, 2020
How AI is Changing the Retail Industry: Role and Impact with Use Cases
Artificial Intelligence (AI) in retail is changing this industry by playing a crucial role in the various key divisions.
Read More →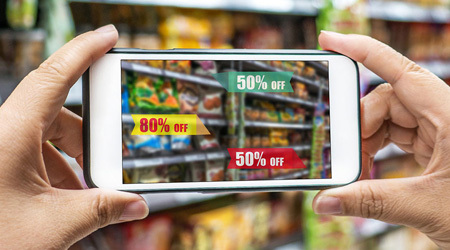
- AI in Retail 21 Oct, 2021
Retail Processes are Being Redefined by Artificial Intelligence
Retail and consumer goods companies have entered a new era of technology innovation, centered on intelligent automation
Read More →