How Training Data used in Autonomous Vehicles Industry
By Anolytics | 04 August, 2022 in Self Driving | 3 mins read
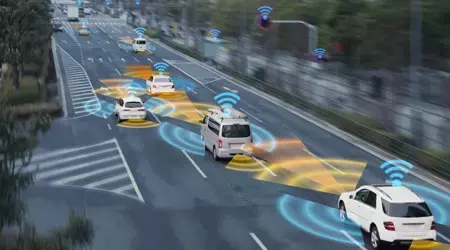
Autonomous cars are expected to transform transportation into the automobile industry’s holy grail. A few years ago, there was an unmatched buzz surrounding driverless vehicles. What happened? Where is the breakthrough in autonomous cars that so many businesses claim we will have by 2021?
It turns out that building a self-driving automobile is significantly more difficult than anticipated. This might be because of lack of training data for autonomous vehicles.
So let’s look at where we stand with self-driving vehicles, why developing them is one of the most challenging problems we face today in terms of training data, and what we can do to change that.
Where do Autonomous Vehicles Currently Exist?
Autonomous cars have a lot of potential since they will revolutionize our highways and make driving safer. After all, statistics indicate that more than 90% of traffic collisions are the result of human mistakes.
Many automakers had significant intentions to have fully autonomous commercial cars on the road in the following few years back in 2015 or 2016, but those targets have long since been surpassed. Although it was an exciting period for the business, the hype had already outpaced the truth.
Why Is It So Difficult to Build an Autonomous Vehicle?
Ultimately, the issue boils down to how difficult it is to develop a completely autonomous vehicle that can operate in any situation. Companies have had to postpone timeframes, sell off autonomous operations, and change their strategy since it is considerably more complex than automotive experts had anticipated. Let’s discuss what makes this kind of endeavor so challenging:
1. The World Is Complicated, for One. Autonomous cars must go through a complicated environment that includes many roads, street signs, people, other vehicles, buildings, and more. Therefore we need high-quality training data for self-driving cars.
2. People can’t be predicted. These cars must be able to anticipate human behavior, which is somewhat unpredictable, and understand their driver.
3. Technology is Pricey. Autonomous cars employ hardware (such as cameras, LiDAR systems, and RADAR) mounted to collect data from their surroundings and aid decision-making. But in order to deliver the kind of thorough data cars require, this gear still has to be significantly improved. Additionally, it isn’t particularly economical.
4. Quality training data for autonomous vehicles. Autonomous cars must be prepared for any scenario (for instance, harsh weather like snow or fog); it is incredibly challenging to foresee every situation a vehicle can experience.
5. There isn’t any room for error. Given how directly they affect the safety of drivers and passengers, autonomous cars have a life-or-death use case. These systems need to be very precise.
Data Is the Secret
Looking at their origins is necessary to address the problems mentioned above. And in order to achieve so, we must comprehend how a self-driving automobile operates.
These vehicles use artificial intelligence, particularly computer vision models, which let the automobile “see” the environment around it and then make judgments based on what it observes.
As we discussed, cameras, LiDAR, RADAR, and other forms of sensor data are collected from hardware on the vehicle and utilized as input for the models. For instance, an automobile must have previously seen sensor data depicting a pedestrian on the road to respond to one.
In other words, it has to be trained on data that includes examples from every event and circumstance. Considering the various possibilities, you encounter when driving, you can see how this results in a lot of training data for autonomous driving.
Data Annotation Types in autonomous Industry Include:
1. 2D Bounding Boxes
2. Lane Marking
3. Semantic Segmentation
4. Video Tracking Annotation
5. Point Annotation
6. 3D Object Recognition
7. 3D Segmentation
Data Partners Can Be Useful
Given the significant increase that automotive projects demand, many businesses have previously relied on various providers to assist them in gathering and preparing the data necessary to train their models.
In practice, it is preferable to identify a single data partner with the knowledge and resources to help throughout the project. You can grow projects to many sites and reach the most fantastic accuracy required for AI-powered autonomous cars with the aid of a trustworthy data annotation partner.
Businesses may gain a competitive edge in the autonomous vehicle market and, more widely, the automotive industry if they can locate a reliable image annotation partner for autonomous vehicles to fuel their requirement for autonomous vehicles.
We at Anolytics, rely heavily on the human-in-the-loop workforce for building training data for self-driving cars. We provide flexible involvement in the labeling loop since we depend on the labelers to react fast and adjust workflow as needed depending on the model testing and validation phase.
please contact our expert.
Talk to an Expert →
You might be interested
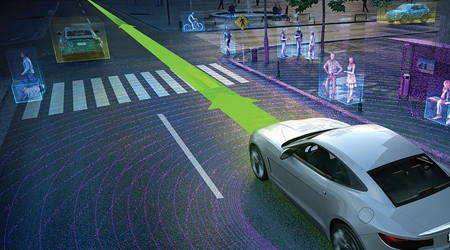
- Self Driving 30 Jan, 2020
How to Improve Computer Vision in Autonomous Vehicles using Image Annotation Services?
Self-driving cars need more precise visual training to detect or recognize the objects on the street and ride in the rig
Read More →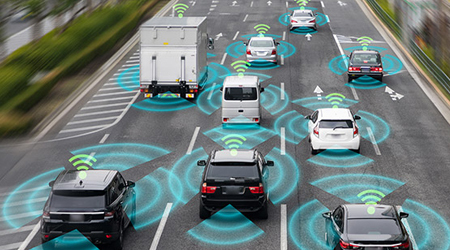
- Self Driving 12 Aug, 2020
Best Challenges with Autonomous Vehicles: The Self-Driving Car Problem
Autonomous vehicles, especially self-driving passenger cars are like a dream when it will become come true. Yes, I’m t
Read More →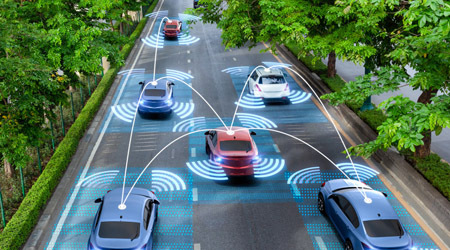
- Self Driving 17 Sep, 2021
The Safety Framework in Autonomous Cars
Ever wondered how the vehicular safety framework has been defined in autonomous vehicles?Till now we have heard of the A
Read More →