How Data Quality Contributes to AI Success
By Anolytics | 25 January, 2023 in Data Annotation | 5 mins read
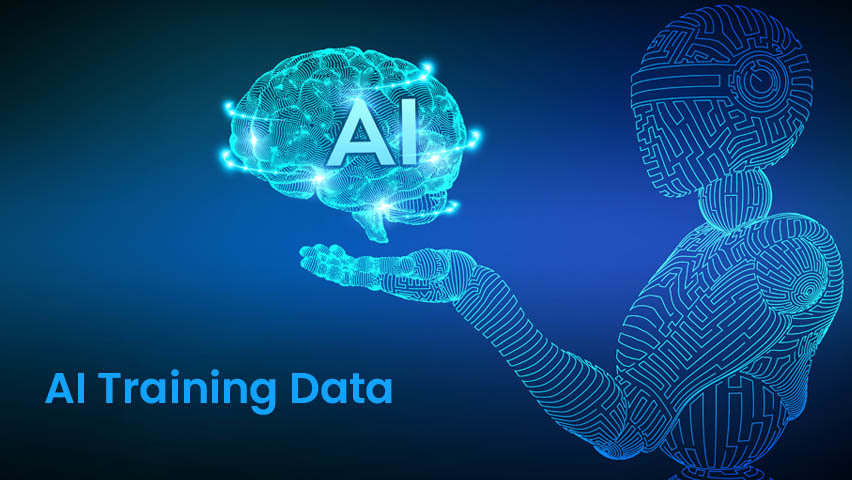
The quality of datasets plays an important role in the functioning of artificial intelligence (AI) in machines and applications. Acquisition and validation of data annotation are the most challenging parts of a data-intensive AI application. It is highly valuable for an ML (machine learning) model to have high-quality data, which makes it capable of making better and faster decisions.
Data Quality is Proportional to the Performance of AI Models
The majority of efforts in developing a machine learning model go into collecting and preparing data. Exactly why? It’s because you can only train machine learning algorithms with data that’s good. A bad algorithm won’t be able to find the right solution if it’s given bad, irrelevant, or faulty data. It’s also true the other way around. High-quality data will help a model perform better and solve more complex problems if everything else is done right.
A model’s performance is directly related to data quality, how much time and effort will be required to train it, and how the project will progress in general. Hence, performing data quality checks is crucial to filling gaps, removing duplicate information, and fixing any other anomalies, training data experts at Anolytics, of the leading image annotation companies, believe so. Although doing everything correctly may take some time, it will save the data team an enormous amount of time in the long run by preventing potential problems from occurring during the course of the project.
Qualitative Aspects of AI Training Data
It is critical that the data used to build AI models be of high quality. Models that are based on incorrect data can only succeed in the real world if they are up to scratch. Good data quality, however, can lead to error-free machine learning and, thus, accurate and better-performing AI models.
The quality of data can be impacted by a variety of aspects, including:
– Choosing data from a trustworthy and reliable source is more likely to result in high-quality data.
– The methods used to collect data
– How the data is cleaned and processed
– Categorizing data based on their usability for testing, validating, and training
The quality of data and, therefore, the performance of the AI model is determined by each of the above aspects. For AI models, data quality is influenced by many factors. The amount and scope of the training data used to train a model can significantly impact its accuracy. It is also important to consider the features of the dataset and the extent to which they were accurately extracted from the source.
Aspects Anolytics Considers to Determine Data Quality
In order for a machine learning model to work, data is essential. However, what determines the quality of the data determines the functionality of a machine learning model, i.e., how specific it is to the process and utilitarian for the model it has been developed for. For this reason, we at Anolytics, an industry leader in image annotation outsourcing, devote more than half of our time to improving the training data.
1. Achieving Accuracy
Data that is of high quality tend to be more up-to-date and accurate. AI models are judged on the accuracy of their predictions of certain values based on the algorithm. A model’s accuracy will depend on its purpose, how it was trained, and how it was used. We combine human expertise and AI intervention in our data annotation process at Anolytics in order to make sure that the AI training data we develop at Anolytics is accurate.
2. Keeping the Data Integral & Complete
An AI model’s workability depends on the data’s integrity and completeness. It’s more likely to have high quality when the data is complete. Data with complete information is the best way for AI models to make predictions since this way, they have enough information to make decisions and predictions. A company’s AI model won’t be able to predict customers’ preferences if they don’t have information about how its customers use their product. Our aim at Anolytics is to keep the data as integral and complete as it can be so as to make the AI model more comprehensive.
3. Maintaining consistency
It’s more likely that data will be high quality if it’s consistent and error-free. AI models require a set of inputs that are consistent with each other for them to make decisions because they must make decisions based on a consistent set of inputs. In this case, a customer service artificial intelligence model would be a good example. It is also more reliable and predictable if the inputs and outputs are consistent.
4. Maintaining Timeliness
A high-quality dataset will be up-to-date and reflect current circumstances. By taking in new data as it comes in, machine-learning models can make more accurate predictions. To be able to use image annotation for deep learning or in an automated learning model, the data must be developed and delivered in a timely manner.
As a reliable image annotation company that has been in the AI space for more than half a decade, Anolytics knows the value of timely data analysis and acquisition for its clients’ machine learning models. With a large pool of in-house data annotation and labeling experts, Anolytics is able to deliver data in a timely manner without sacrificing accuracy.
5. Validating for Deployment
The validity of data refers to the level of reliability and accuracy provided by a data set. It is possible, for example, that you will say blue even if you actually like green if someone were to ask what your favorite color is. A friend may appear to be 3 feet away when they are actually 20 yards away when you are walking down the street. A model will give incorrect results if the data is not valid, which is crucial to machine learning.
6. A Commitment to Maintaining Uniqueness
To prevent overfitting, it is important to have unique data for a dataset. A model that has been overfitted will not generalize properly if it is taught with too much or incorrect data. A model takes into account any variations arising from different individuals in your dataset by using unique data.
Also read: Valuable Insight into Image Annotation: What & How?
Quality Control vs. Quality Assurance
Ensure the accuracy, consistency, and completeness of data through data quality control (DQC). Using this method, AI systems can gather more accurate data. In order to accomplish this, an outlier detection model is created.
Assuring data quality meets the needs of users is referred to as data quality assurance (DQA). Our process of ensuring that training machines have high levels of accuracy and reliability for AI datasets is known as training machine learning models. A model’s accuracy is a measure of the probability that a model will produce the expected results when given a query, which is an important factor in ensuring data quality.
Also read: A Detailed Guide to Data Annotation & Labeling for AI
Final Thought
The ability of AI to make accurate decisions can be hampered without high-quality, diverse data. Data quality is essential to ensure AI’s data is reliable and meaningful. Your models can be accurately trained by diversifying and standardizing your data sources. Furthermore, you can improve the performance of your AI applications by ensuring the accuracy of your training datasets.
Anolytics, being a credible image annotation company, has been promising a model-agnostic training data platform to AI enterprises across the globe. We boast reasonable image annotation pricing for timely data delivery. Our teams review data logs to determine if issues exist before their input data tables are affected. As a result, reasonable image annotation pricing saves you money, freeing up your resources for other important tasks during the development of AI models.
please contact our expert.
Talk to an Expert →
You might be interested
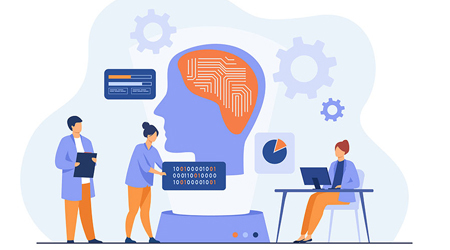
- Data Annotation 02 Aug, 2019
Five Reasons to Outsource Your Data Annotation Project
Artificial Intelligence (AI) and Machine Learning (ML) development is mainly rely on training data sets, that helps the
Read More →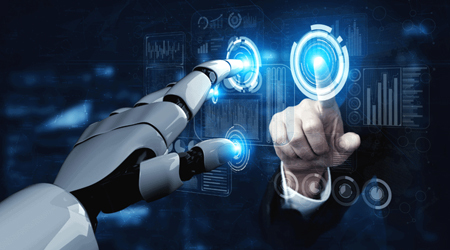
- Data Annotation 17 Dec, 2019
Why Data Annotation is Important for Machine Learning and AI?
Data annotation is the process of making the contents available in various formats like text, videos and images, recogni
Read More →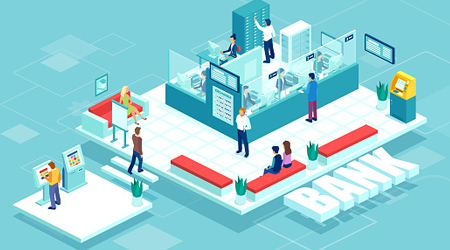
- Data Annotation 13 Oct, 2020
Top Most Data Labeling Challenges in Annotation Companies
Data labeling is not a task, it requires lots of skills, knowledge and lots of effort to label the data for machine lear
Read More →