What is the Best Challenges with Annotate 3D Point Cloud Data for LIDARs?
By Anolytics | 18 January, 2021 in 3D Point Cloud | 4 mins read
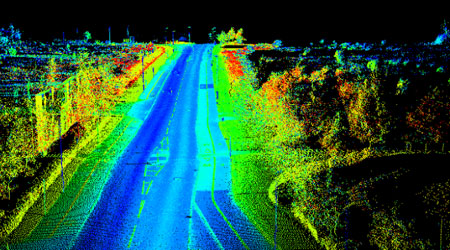
3D point cloud data is one of the most complex types of machine learning data used to generate the most useful training datasets for autonomous vehicles. LiDAR data is annotated with 3D point cloud annotation to make the objects more precisely detectable to a self-driving car.
LiDAR & 3D Point Cloud Annotation
LIDAR is a kind of remote sensing technology using light in the form of a pulsed laser combined with other data recorded by the airborne system to generate precise, three-dimensional (3D) information about the shape of the Earth, its surface characteristics, and various objects visible there.
A bunch of LIDAR data put into the deep learning algorithms, helps autonomous cars detect and measure the distance of objects precisely. Dodges in the making the LIDAR datasets can give inaccurate information to the car resulting in wrong interpretation increase the chances of collisions.
Also Read: LIDAR Sensor in Autonomous Vehicles: Why it is Important for Self-Driving Cars
3D point cloud annotation is the most useful as well as challenging image annotation technique used to generate data that helps deep learning algorithms to better understand the surroundings. The LIDAR data can be made useful for autonomous vehicles only when it is annotated with 3D point cloud data.
3D Point Cloud Data
The point cloud is like a set of pints in space, while a 3D point cloud is a collection of data point equivalents to the real-world scenario in three dimensions. The 3D point cloud the data is defined by its own position and color and points can then be rendered as pixels to create a highly accurate 3D model of the object.
Point clouds can easily describe objects measuring from just a few millimeters to objects as large as buildings, trees, and even entire conurbations.
And to collect geographic point cloud data LiDAR sensing scanners are used that can gather the accurate mapping of the objects. LiDAR can shoot pulses of light up to a million pulses per second that bounce off a surface and return to the sensor that calculates the time it took for the ray of light to return and which direction it came from.
The entire process ultimately creates a point cloud map of the scanned surroundings to store every detail down to the last millimeter, while capturing all the different contours and shapes. But there are few shortcomings with LiDAR and using the same for gathering such data.
Limitations of LiDAR Data
Just like any other advanced technology, LiDAR has few limitations that create problems not only for annotators but also for the AI researchers and machine learning engineers using the LiDAR into various other fields.
LIDAR Limitations:
- LiDAR needs visible to access real objects.
- Reflective surfaces can create problems for the laser.
- It’s not suitable for capturing moving objects.
- Bad weather can interrupt data collection.
- Investment in LiDAR is very costly for researchers.
Though, LiDAR has its limitations but still it is one of the best sensing technologies providing more useful information to autonomous cars. The LiDAR data becomes more useful when it is labeled with 3D cloud annotation.
Applications of 3D Point Data
The application of 3D Point Data is mainly into autonomous vehicles but now other computer visions related AI models like drones and robots are also using LiDAR for better visual perception. Before we discuss the challenges with 3D point cloud data, we need to understand its applications.
Self-driving Cars or Autonomous Vehicles
3D point cloud data is most useful for autonomous vehicles that need to understand their environment and perform simultaneous localization and mapping in real-time in order to keep moving in the right direction avoiding obstacles.
Also Read: Why Self-driving Cars Taking Too Much Time: Challenges of Autonomous Vehicles
In Other LiDAR-based Applications
The applications that need LiDAR-based accurate data also need 3D point cloud to make such data more precise. LiDAR is mainly known for gathering the measurements of objects with a tremendous degree of detail.
If your AI model is going to be used in the real-world where the accuracy of gathering such data is practically associated with life and death situation, then you need to rely on LiDAR for capturing the tiny details.
To Explore Dense Forest & Dangerous Hidden Locations
Have you heard about the sprawling maya network discovered under guatemala jungle?, that has been discovered with the help of LiDAR sensors on aerospace that mapped the dense forest and discovered the subtle signs of the archaeological wonder.
This hidden ancient place has amazed the archeologists, especially about the potential of LiDAR technology. Yes, LiDAR can be used in various other dangerous situations the places that are not safe for human mapping manually.
But robots or drones with LiDAR sensors can reach such dangerous locations and scan or generate useful data helping humans to navigate safely at such risky places and avoid mishaps. Natural disasters, unknown hidden dense forests and dark caves are the best examples of such dangerous locations.
Challenges with 3D Point Cloud Data
The data generated through LiDAR is not only useful but also complicated to understand with normal vision. Hence, it is labeled with 3D point cloud annotation to make the objects detectable to deep learning algorithms. But dealing with 3D point cloud data is also not easier, as there are few challenges you need to know.
Massive Size of Files
The file size of LiDAR generated data is so huge that you need high configuration devices to store, manage, and utilize such data. Though, there are many publically available 3d point cloud datasets but downloading that can hang your computer.
Complex User Interface
As you already know we have to deal with 3D instead of 2D, so you need a more complex user interface which quite comprehensive and usable. And achieving this task is challenging, especially for the annotators who are dealing with such data.
Highly Costly Sensors
Point loud data is gathered through various sensors like LiDAR or other lasers based technologies that are very expansive. Though, in the future, the cost might come down due to more innovations and optimization in manufacturing and producing such sensors.
Although, more affordable options are available for researchers expansive sensors can provide the details that low-cost devices can’t. But if you want to train your deep learning model with a better quality of data, you need to go with the superior quality of sensors and training data.
Also Read: Top Data Labeling Challenges Faced by the Data Annotation Companies
Better quality data here means the computer vision training data you need to train your machine learning or deep learning model. Anolytics right here doing the same job with a team of dedicated and highly skilled annotators for annotating all types of data including LiDAR.
For 3D point cloud annotation, our enriched experience and expertise in image annotation services will give an advantage of getting high-quality training data for self-driving cars, robots, and drones. It offers a human-powered AI-assisted data labeling service to produce world-class training datasets.
Also Read: Why Human Annotated Datasets is Important for Machine Learning
please contact our expert.
Talk to an Expert →
You might be interested
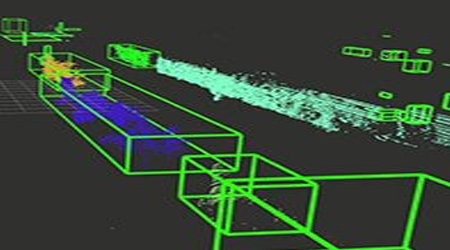
- 3D Point Cloud 14 Dec, 2021
Scenario identification is the result of 3D point cloud segmentation
Data can take many different forms. Images are widely used in the processing of visual information. Images are made up o
Read More →